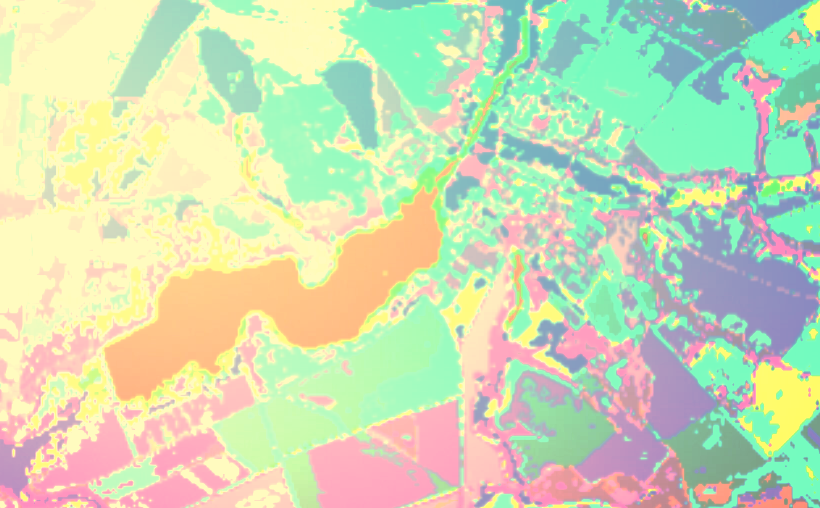
When we perform image classification, we try to get the best possible result. Despite this, often the result of the classification, although good, is not perfect. And no classification procedure settings can improve the accuracy of class recognition. In this case, it is possible to improve the result using post-processing algorithms. They include procedures for combining […]